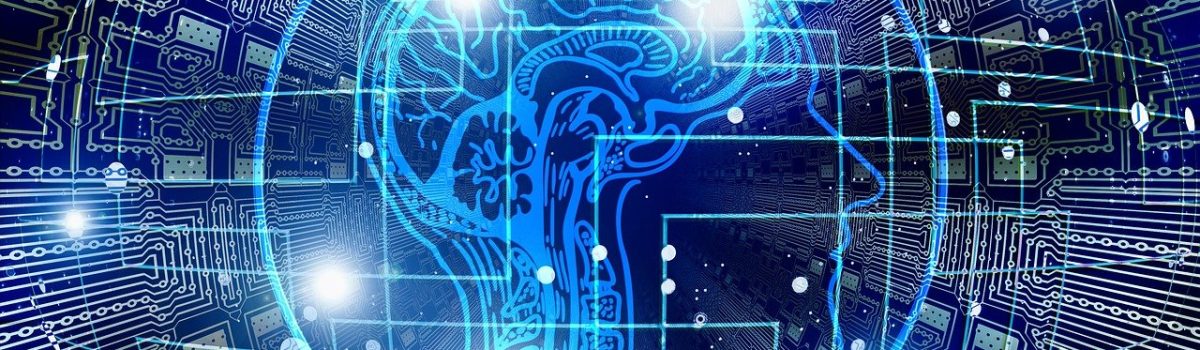
Minimal Viable Data Strategy for Early-Stage Startups: Leveraging AI and Big Data Without a Formal Corporate Structure
Abstract
Startups today face a lot of change, especially with artificial intelligence (AI) and big data at play. These new tools can help, but they can also be tricky without a solid company structure. This paper introduces a simple plan called the Minimal Viable Data Strategy (MVDS). It’s designed for new businesses that want to use data to make smart choices while staying flexible. We suggest a clear way to collect, analyze, and use important data. This includes looking at how users behave, spotting market trends, and keeping an eye on competitors. By using affordable AI tools, startups can handle data without needing big, expensive systems. Through examples and real-world studies, we show how MVDS works in different startups. It helps companies find the right product for their market and use their resources better. Our research shows that a smart, straightforward approach to data helps startups grow faster and tackle the unknowns of starting out. This study adds to the conversation about using data in business. It provides a helpful model that fits the changing needs of new companies.
Introduction:
In today’s fast-moving digital world, new startups have their own set of challenges and chances. A key part of starting a successful business is how well you can use data. Unfortunately, many new companies don’t have the resources or structures of larger firms to manage data effectively. This paper suggests a Minimal Viable Data Strategy (MVDS) specially designed for early-stage startups. It focuses on using AI and big data to make better decisions, connect with customers, and spark new ideas, all without needing a fancy corporate setup. Big data and AI have changed how businesses operate. They give access to huge amounts of information and smart tools to analyze it. Startups are usually quick and creative, thriving in uncertain and fast-changing situations. But traditional data management can slow them down. The MVDS framework simplifies how startups gather and use data in a cost-effective and flexible way so they can stay quick on their feet and make the most of data. A Minimal Viable Data Strategy starts by pinpointing what data is essential for the startup’s goals. It focuses on useful insights instead of trying to collect everything. This means paying attention to relevant sources like customer feedback, market trends, and key performance indicators. Startups can use AI tools for analyzing data. Machine learning and predictive analytics can help them find valuable insights even from small amounts of data, which helps them make smart choices. The MVDS also highlights the need for a data-friendly culture in startups. By embracing a mindset that values trying new things, learning from mistakes, and continuously improving, new companies can encourage innovation. This change not only helps teams use data better but also sets the stage for developing more advanced data strategies as they grow. A Minimal Viable Data Strategy gives early-stage startups the tools they need to tackle the challenges of today’s data-driven world. By using AI and big data simply and smartly, these businesses can improve their operations, provide better experiences for customers, and drive steady growth. This paper discusses the elements, benefits, and practical uses of MVDS, offering guidance for startups looking to make informed decisions in a tough market.
Core Concept and Rationale
A Minimal Viable Data Strategy (MVDS) is all about finding out what data a startup really needs. It’s a simple way to gather, check, and use the data that helps run the business. The aim is to make sure the data strategy is strong enough to give useful insights while still being flexible as the company grows. Startups usually work with limited resources and need to be quick and adaptable. That’s why the MVDS focuses only on the most important data. This way, startups can make quick decisions without the confusion that comes from huge data strategies used by larger companies that have big teams and budgets. There are many good reasons for going with a Minimal Viable Data Strategy, especially for new businesses. It fits right in with lean startup principles, promoting fast testing and learning. By gathering the least amount of necessary data, startups can quickly test their ideas and change direction based on what they find out. Startups often deal with a lot of uncertainty and change. Rigid data processes can slow them down. An MVDS helps keep them quick on their feet, adjusting their data collection as they learn more about their customers and the market. Startups have access to great tech tools. They can use AI and big data without needing a ton of infrastructure. Cloud solutions and open-source tools allow even the tiniest startups to use data for growth. Another big point of the MVDS is focusing on insights that lead to real action. Startups should look for data that matters and can be measured. Identifying key performance indicators (KPIs) that match their goals is key. By concentrating on useful data, startups can make smart decisions based on what really counts. Adopting a Minimal Viable Data Strategy helps create a habit of making data-driven decisions right from the start. When data is part of the decision-making process, it encourages a culture that values facts over guesses. This foundation is crucial as the business grows, helping to keep data at the heart of the strategy.
Regulatory and Ethical Dimensions
A Minimal Viable Data Strategy is all about keeping things simple. It helps startups use data without getting lost in complexity. This is super helpful in the beginning when teams are small and money is tight. The main goal here is to quickly check if business ideas work. The strategy focuses on three main areas: collecting data, managing it, and using it properly, all while following the rules and being ethical.
Startups should find the data that matters most to their business. This can include info from customer chats, market research, competition, and social media. Using tools to automate data collection can save time. But, it’s important to follow rules like the GDPR and CCPA to make sure personal data is handled correctly.
After collecting data, managing it well is key. Startups need to create a simple data system that is easy to access and analyze. They should pick the right storage options, like cloud solutions, and set up rules to keep data safe and accurate. As they grow, they can improve their management practices, but starting with a solid base is essential.
The goal of any data strategy is to turn data into useful insights. Early-stage startups can use AI to analyze their data. This could help with things like figuring out customers, predicting trends, or spotting patterns. However, it’s crucial to use data responsibly, ensuring that decisions are fair and respecting user privacy. Startups face big challenges when it comes to following the rules, especially if they work with sensitive data. Knowing the laws around data use is essential to avoid fines and keep a good reputation.
Startups need to know the rules that apply to them. For example, GDPR has strict guidelines about how to collect and use personal data, needing clear consent from users. If a startup works in different places, they have to comply with various laws everywhere.
Getting clear consent from users is vital. Startups should write understandable privacy policies explaining how their data will be used. Being transparent builds trust with users and meets legal needs.
If a data breach happens, startups need a plan. This should include notifying affected users and regulatory bodies within a set time. Having a clear response plan shows commitment to protecting user data and meets legal requirements.
Startups should appoint someone to oversee data protection laws. Regular checks can help find risks and areas to improve.
It’s not just about following the law. Ethical issues are also important in how startups handle data. They need to think about the impact of their data practices and aim to be responsible.
AI can unintentionally carry bias if not designed right. Startups should focus on fairness, using diverse data sets and regularly checking their algorithms for bias. This is especially crucial in areas like hiring and lending.
Protecting user privacy is a must. Startups should only collect the data they really need and have strong security to keep that data safe. Giving users the choice to opt-out of data collection helps build trust.
Using AI wisely is important. Startups should be clear about how their AI makes decisions and what data it uses. This helps users understand the process better.
Startups can use their data practices to help society. This can involve using data to improve health care or education. By aligning their data strategies with social causes, startups can create positive impacts for both their business and the community.
Technological Approaches
A Minimal Viable Data (MVD) strategy is all about knowing that not all data is useful. Startups should focus on collecting and analyzing the data that really matters for their business goals. They don’t need a mountain of data from everywhere. Instead, they should aim for the most useful datasets that can help them make quick decisions. This way, they can respond to changes in the market without wasting time and resources. One smart way to do this is by using cloud storage and processing. Services like Amazon Web Services, Google Cloud, and Microsoft Azure let startups store and analyze data without spending a lot upfront on technology. These platforms come with various tools for data handling, machine learning, and analysis. This helps startups use big data without getting bogged down by complicated tech requirements. Cloud solutions also allow startups to be flexible. They can easily change how they collect data or try new ways to analyze it. This adaptability is key for startups that need to stay quick on their feet in a competitive world. Startups can also benefit from automated data collection and analysis tools. They can use web scraping tools, APIs, or platforms like Zapier to gather data without doing everything by hand. Automation makes life easier for team members, so they can focus on understanding the data better. AI can enhance an MVD strategy by spotting patterns in data, predicting trends, and making quick predictions based on past data. Startups can use ready-made machine learning models from platforms like TensorFlow or Scikit-Learn without being data experts. This opens up data access for everyone in the team, so everyone can help make smart choices based on data. AI tools can also help startups find the most important performance metrics to focus on. By keeping an eye on just a few key metrics, they can avoid drowning in data and instead look for practical insights. Tools like Google Analytics or Tableau can create clear dashboards that show important trends, helping startups track their success and make changes when needed. Using open-source technologies can also help startups. Programs like Apache Kafka for data streaming or PostgreSQL for database management cost little to integrate into their tech setup. Open-source tools cut costs and encourage teamwork and innovation. Startups can tweak these tools to fit their specific needs. Data governance is another important piece. Even small startups need to have clear rules for how they handle data. Having guidelines helps protect against privacy issues. Startups should use tools that offer built-in security features, like data encryption, to safeguard sensitive info. By prioritizing data governance, startups can earn trust with their customers and avoid legal problems. Collaboration tools like Slack or Trello are also key to making an MVD strategy work. These platforms help team members communicate and manage projects, making sure everyone is on the same page about data efforts. Regular meetings and idea-sharing sessions can create a culture that values data, encouraging everyone to contribute. Startups must keep checking and improving their MVD strategy. As things change, so will their data needs. It’s important to have a system where insights from data help refine future collection methods. Staying updated on new technologies and trends in data will help startups keep their strategies relevant and effective.
Cloud-Based AI and Analytics
A minimal viable data strategy focuses on the main things you need to collect, analyze, and use data efficiently. For new startups, the aim is to learn a lot while using fewer resources. The key is to set up a system that helps you make quick decisions based on what the data shows. This helps you handle the unknowns in the market better. The main parts of a minimal viable data strategy include collecting data, analyzing it, and making decisions based on what you find. Startups should gather the data that really matters for their goals. They need to analyze this data well and use the findings to guide their choices. By keeping it simple, startups can save money and focus on learning and adjusting as they grow. Cloud-based AI and analytics are super helpful for startups trying to use a minimal viable data strategy. Cloud services give flexible tools to manage lots of data without needing a big upfront cost for devices. Plus, with AI technology, startups can use smart systems to find insights in their data quickly.
Here’s how cloud services help:
Cloud platforms let startups easily adjust their resources. This is great for new companies that might have changing data needs. They can start small and grow as needed, only paying for what they use.
Setting up traditional data systems can be really expensive. Cloud options usually let you pay for what you use, which helps keep costs in check. This means startups can spend money on other important things like product development.
Cloud tools let team members access data from anywhere. This encourages teamwork and makes it easier to make decisions based on data. This is especially important for startups with remote teams or when quick responses are needed.
Many cloud services work well with different data sources. Startups can connect their CRM, marketing tools, and other systems to one place, making it easier to collect and analyze data.
Cloud AI tools can look at data on a large scale, finding patterns that manual checks might miss. Startups can use these insights to improve operations, enhance customer experiences, and craft better marketing plans.
To implement a minimal viable data strategy, startups should consider these steps:
Clearly outline your business goals. What questions need answers? What issues do you want to fix? This helps narrow down what data to collect.
Figure out where to get your important data. This might include customer interactions, social media, website stats, and sales figures. Focus on data that ties back to your goals.
Pick cloud-based tools that fit your needs. Options like Google Analytics, AWS Analytics, or Microsoft Azure can help analyze data effectively.
Even without a big team, having basic rules for data management is key. Decide who will collect and analyze the data. Keeping data quality and security in check is important too.
Keep testing and adjusting your strategies based on your data analysis. Being flexible helps you adapt to market changes.
Promote a culture that prioritizes data-informed decisions. Train your team to understand data and use insights in their work.
Regularly check how well your data strategy is working. What insights are you getting? Are they leading to good choices? By reviewing the impact of your data, you can improve your strategy over time.
Off-the-Shelf Tools
A minimal viable data strategy (MVDS) is a simple way for startups to handle their data needs. It helps them focus on what matters to reach their goals. Big companies might have the money and staff for complex systems, but startups often don’t. So, MVDS is all about being quick and straightforward. With this approach, startups can make smart decisions based on data without feeling overwhelmed by details. Here are the main parts of a minimal viable data strategy:
Startups need to know exactly what they want to achieve. Are they trying to make customers happier? Improve how they run things? Or offer better products? Having clear goals helps them decide what data to collect and analyze.
Startups should only gather the data they really need. This can include things like customer info, sales numbers, website stats, or social media activity. It’s important to focus on quality data that can lead to real insights.
After collecting data, startups should analyze it for insights. This doesn’t have to be hard. They can use easy-to-use tools that help visualize data and spot trends. This helps in making informed decisions.
The MVDS is flexible. Startups should be open to testing ideas, getting feedback, and making changes to their data strategy. This way, they can quickly adapt to feedback and changes in the market.
Even though the focus is on simplicity, startups should also think about growth. As they expand, their data strategy should change to handle more data and complexity.
AI and big data are useful tools for startups. They can help boost innovation and growth. By using these technologies, startups can better understand their customers, streamline their operations, and offer personalized experiences. AI can help startups save time and improve decision-making. Here are a few ways AI can fit into a minimal viable data strategy:
Startups can use AI to look at past data and make predictions about the future. For instance, by reviewing past sales, AI can help guess future demand. This helps startups manage inventory and cut costs.
AI can help startups group their customers better. By analyzing data, they can find different groups of customers. This makes targeted marketing and personalized experiences easier.
Adding AI chatbots can improve customer support without needing a big team. Chatbots can answer common questions, allowing human staff to tackle tougher issues.
Startups can use AI tools to create content, from ads to social media posts. This not only saves time but also keeps messaging consistent.
Data Minimization by Design
Big data and AI are changing how businesses work. Startups, in particular, can take advantage of these tools to grow. But with so much data out there, it can feel overwhelming. A Minimal Viable Data Strategy helps startups figure out what data they really need. This way, they can focus on the essentials, cutting down on waste.
Data Minimization by Design
What’s the Idea?
Data Minimization by Design means that businesses should gather only the data they truly need. This approach helps startups who may lack the resources to handle a lot of data. By focusing on what matters, they can work more efficiently, gain user trust, and follow rules like GDPR and CCPA.
Why Minimize Data?
Collecting less data means less money spent on storage and processing. This saves startups money for other important things.
When startups limit data, they can zero in on key metrics that really matter, instead of getting lost in unnecessary info.
With stricter data protection laws, minimizing what they collect helps startups avoid legal issues and builds trust with customers.
With fewer data points, the quality goes up. There’s less noise, which makes it easier to find valuable information.
By focusing only on what’s useful, startups can work quicker and make better decisions.
How to Use a Minimal Viable Data Strategy:
Startups need to know what problems they want to solve. This will help them identify the data they actually need.
Identify important performance measures that match their goals. This means picking a few metrics that give good insights without overloading the team.
Data gathering should be straightforward. Startups can use surveys, feedback, and analytics to collect only what’s necessary. Setting up processes for high-quality data is key.
Smart use of AI can make a Minimal Viable Data Strategy work better. Startups can analyze smaller datasets to find patterns. AI tools can also help handle data processing.
Startups should foster a culture where decisions are made based on data. It’s important to appreciate the benefits while understanding data limits.
Data strategies need to change over time. Startups should regularly check how well their data collection and analysis are working so they can adapt as needed.
Think About Ethics:
While building these data strategies, startups should think about ethics. Minimizing data not only helps with legal rules but also helps build trust with consumers. They should:
Clearly explain why they collect data.
Protect the data they gather.
Give users control over their data, letting them opt-out of non-essential collection.
By focusing on what really matters, startups can make better choices and stay on the right side of data practices.
Benefits and Challenges
The Minimal Viable Data (MVD) strategy helps startups keep things simple. Instead of trying to collect and manage tons of data, startups focus on gathering just what they need. This way, they can make smart decisions and adapt quickly. Since many startups have tight budgets, a clear data plan can really help them grow over time. By identifying only the essential data points, they can use their resources more wisely.
Here are some benefits of an MVD strategy:
Startups often run on limited budgets. By only collecting necessary data, they can cut costs on data storage and analysis tools. Instead of buying expensive software, they can use free or cheaper options.
Startups need to move fast. An MVD strategy allows them to quickly pull insights from the data without getting lost in complicated systems. This speed helps them change their plans based on market feedback, boosting their chances of success.
With an MVD strategy, startups learn to pinpoint the most vital data for their growth. This focus helps them track important metrics without getting distracted by unnecessary data.
Startups can use minimal data to test their ideas and see what works. By gathering just enough information, they can check customer reactions and market trends without spending too much upfront.
Many startups don’t have big teams to handle data rules. Keeping a small data set makes it easier to follow laws about privacy. This way, they can stay safe without getting stuck in heavy regulations.
A focused data strategy helps startups understand their customers. Analyzing key information can reveal patterns and preferences, helping them serve their customers better.
Even though MVD starts small, it sets a solid base for future growth. As the startup gets bigger, they can expand their data practices.
MVD encourages creativity. Startups can test new ideas using their data, which leads to better products and services.
Startups can team up with others to share insights. Working with local schools or data organizations can help them learn to use data effectively without huge costs.
With modern tools, startups can turn small data sets into useful insights. Machine learning can help them spot trends and patterns, allowing for better decision-making.
Yet, there are challenges that come with an MVD strategy:
It can be tough to know which data is truly important. Without clear goals, startups might end up collecting too much or too little information.
Some startups might not have team members who are good with data. This can make it hard to collect or analyze the right info. They might need to train their people or hire experts, which can complicate things.
Startups often use different tools for various tasks. Bringing these tools together can be hard. If they don’t integrate well, it can hurt data flow, making analysis difficult.
While starting small can be good, they still need to plan how to grow their data collection as the business expands. This means regularly updating their strategy, which takes time.
If they rush, they might collect poor-quality data, which can lead to wrong insights. Startups need to check their data for accuracy.
Startups have to follow data laws, and mistakes can lead to serious issues. They must keep their data practices in check to avoid legal problems.
Limited time and budget can make implementing a data strategy tough. Startups must find the right balance between daily operations and data needs.
Some team members might be hesitant about using data for decisions. Startups need to help everyone see the value of a data-driven approach.
The startup world is always changing, and keeping a focused MVD strategy can be difficult. Startups may need to adapt quickly as their needs evolve.
While technology helps with data, issues like software failures can create problems. Startups must be ready for tech challenges that could disrupt their operations.
Not every data initiative brings quick results. Team members may expect fast success, which can create frustration. It’s important for leaders to set realistic timelines.
Startups must think about ethics when using data. Setting clear guidelines for responsible data practices is key to building trust.
Using a Minimal Viable Data strategy can really help new startups grow. By focusing on what matters, they can make smart decisions that lead to innovation. While there are clear advantages, challenges also exist that require clever solutions. Startups need to approach data thoughtfully and flexibly. They should keep learning and adapting to maintain the right metrics and comply with data laws. With a well-crafted plan, startups can create a culture that embraces data-driven growth. This creates positive impacts for their business and their customers.
Challenges
In the fast-moving world of startups, data is key. It helps with making smart choices and improving customer experiences. But early-stage startups often don’t have the formal set-ups that bigger companies do. This can create problems when it comes to developing a Minimal Viable Data (MVD) plan. An MVD plan uses AI and big data but aims to keep things simple and cost-effective. While this sounds good, startups face real challenges when trying to put it in place. Minimal Viable Data means focusing on the most important data that startups need to make smart decisions without making things too complicated or costly. Even though this gives a clearer view of data management, many startups struggle because they lack experienced team members, have limited resources, and need to be quick to adapt. Knowing these challenges is crucial for startup leaders and their teams.
Startups often struggle with figuring out what data is truly necessary. It’s vital to find the key performance indicators (KPIs) that match the startup’s business goals. But startups usually operate in uncertain environments, making it tough to identify which data points actually help with decisions. Founders might end up gathering too much data, hoping it will give them a better picture. This can create chaos where important insights are lost in irrelevant info. Defining what “minimal” means can take time and needs team discussions. Startups should brainstorm and use frameworks like Lean Startup principles to identify the data that matters for making smart choices.
Many early-stage startups don’t have data experts on their teams. The skills needed to analyze data are often spread too thin among founders and generalists. This lack of expertise can make it hard to set clear data needs or pull useful insights from the data they collect. Without technical knowledge, startups may find it hard to spot trends or choose the right methods for data collection and analysis. To help with this, startups might consider collaborating with others, checking out online resources, or connecting with local tech communities for guidance.
With many businesses using various software and online tools, integrating data can get messy. Startups often mix different systems like CRM tools and social media platforms. This can create data silos where information is scattered and hard to analyze. When data is spread out, gathering it can take a lot of time. Startups might spend too long trying to pull data together instead of using it for insights. To fix this, startups need to find software that integrates well and consider cloud solutions to make data management easier.
An MVD strategy should be simple. But as startups grow, their data needs will change. A plan that works at first might not meet needs later. For instance, a startup might start with basic customer data but later need more detailed insights as they expand. Startups need to be ready for this change. They should build flexible data models that allow for adjustments. Choosing technology that can grow with their needs is also key.
Good data quality is crucial for making decisions. But in the rush to roll out an MVD strategy, startups might overlook the need for accurate data. Poor data can lead to bad insights and decisions. Important elements include completeness, consistency, accuracy, and timeliness. Startups should regularly check their data for quality. Setting up clear data collection rules and processes can help maintain high data standards.
Stakeholders like investors and customers can have high expectations. These different expectations can complicate implementing an MVD strategy. Startups often feel pushed to show results fast, which can lead to cutting corners in data analysis. To handle this, startup leaders should be clear about their data capabilities and what can realistically be achieved. Establishing milestones and keeping everyone informed can help align expectations.
Every startup has its own culture, and not everyone may be on board with using data for decision-making. Some team members might prefer relying on gut feelings. This can create barriers to using an MVD strategy effectively. To shift mindsets, founders should focus on training and showing the value of data. Encouraging discussions about data findings and celebrating success that comes from data can help build a data-friendly culture.
The startup world is always changing. Customer preferences and market conditions can shift rapidly. This can make implementing an MVD strategy hard, as assumptions made about data may quickly become outdated. Startups need to keep an eye on these changes. Regular feedback and agile data practices can help them adjust their strategies in real time.
As regulations around data privacy grow stricter, startups must follow laws like GDPR. Being small doesn’t mean they can ignore legal requirements about handling data. Non-compliance can lead to big fines and other issues. Startups should consult legal experts to help keep their data practices in check and develop clear ethical guidelines for data use.
Startups typically work with limited budgets, which can impact their ability to carry out an MVD strategy. This affects money and the ability to hire skilled people for data work. To navigate this, startups should look for free or low-cost tools that can help without breaking the bank. Starting small and focusing on essential data projects can build resource availability over time.
Adopting a Minimal Viable Data strategy means change. It can shake things up and may face resistance from team members used to old ways. Managing this change is key to making the transition smoother. To help, startups should involve their teams early in the process and explain why the change is needed. Providing training and recognizing early wins can build support for a data-focused culture.
Startups often need to act fast. But while setting up an MVD strategy, they must find a balance between speed and careful work. Rushing can lead to mistakes and hurt the quality of insights. Teams should set clear processes and timeframes to ensure thorough work. Emphasizing quality over speed can help startups gain meaningful insights without losing their agility.
Starting an MVD strategy is just the first step. Building a data-driven culture takes ongoing effort. Startups must create an environment where data is valued in daily operations. Leaders should show their commitment to using data and encourage collaboration and knowledge sharing within the team.
AI can change how startups analyze data. However, integrating AI into an MVD strategy can be tricky for smaller companies. They might rush to adopt AI without fully understanding their data needs. To avoid chaos, startups should introduce AI step-by-step. Focusing on specific uses that align with business goals can help manage complexity. Competing with larger players can put pressure on startups to act fast. This desire to catch up can lead to shortcuts in their MVD strategy, risking quality. Startups should focus on what makes them unique and play to their strengths. By emphasizing areas that resonate with their audience, they can find their own space in the market instead of mimicking bigger companies.
Future Directions for Research
In today’s world, having a good data strategy is super important, especially for startups. These small companies are known for being quick and innovative. They need to make smart choices fast. They also need to adapt to what customers want. But, many startups struggle because they don’t have the structured setup that larger companies do. This is where the idea of a Minimal Viable Data (MVD) strategy becomes key. It helps startups gather and use data without all the heavy processes. Let’s look at how MVD strategies can help startups grow and what areas need more research. A minimal viable data strategy is all about keeping things simple. It focuses on collecting only the data that matters. This saves time and money. It fits well with the Lean Startup method, which promotes quick changes based on customer feedback. Using AI and big data tools, startups can stay ahead. They can make decisions based on whatever data they have, even if it’s limited. As we think ahead, there are many areas for research that can improve these strategies.
We need to learn how using minimal viable data strategies affects how startups grow. By looking at how these strategies help with gaining and keeping customers, we can see what works best for startups. Most studies look at data strategies for big companies, so there’s a gap when it comes to startups.
AI is a valuable tool for startups aiming to be data-driven. Future research should explore how AI can help automate parts of MVD strategies. This can make data analysis easier and support better decision-making. Checking how AI can clean data and predict customer behavior is worth looking into.
For data strategies to work, the people making decisions need to know how to use data. It’s important to research how well team members understand data and how that affects MVD strategies. We should find out what skills are necessary and whether startups need dedicated data experts or if existing team members can learn what they need.
As startups grow, their data needs change. Research should focus on creating MVD strategies that can grow with the business. This includes best practices for collecting and analyzing data and when to move from a simple to a more complex approach.
Data use comes with ethical responsibilities. It’s crucial to study how startups can bring ethical practices into their data strategies early on. This research can help startups navigate issues like user consent and data security.
Setting clear performance indicators is key to checking how well data strategies work. Future research should identify the right key performance indicators (KPIs) for startups using MVD strategies. This will help assess their success systematically.
How startups can work together on data strategies is another important topic. Collaborating can help startups share insights and improve their data practices. Examining successful partnerships can reveal best practices and challenges.
Understanding customer behavior is crucial. MVD strategies can help track how customers interact with a business. Researching which data points are important can help startups market better and improve customer experience.
We should also look at how startups can include big data into their MVD strategies without making things complicated. This research can show how big data can complement MVD without overwhelming operations.
Data governance is vital for keeping data secure and accurate. We need to explore how startups can implement effective governance while using MVD strategies.
Startups need to be aware of risks to their data. Research should look into potential threats, like data breaches or incorrect handling. Understanding these issues can help startups protect their data.
Telling stories with data is becoming popular. Research should see how startups can use data storytelling to connect with customers and investors better.
The culture within a startup can affect how well they use data. It’s important to investigate how encouraging data openness and readiness to experiment can improve results.
With many startups using MVD strategies, understanding the competitive landscape is important. Research could help map out trends and what works well across different industries.
The Internet of Things (IoT) is increasingly affecting data collection. Future research should explore how startups can use IoT devices within their MVD strategies to gather useful insights.
Conclusion:
In today’s startup scene, having a Basic Data Plan (BDP) is super important. It helps new businesses use AI and big data even when they don’t have a lot of formal processes set up. A BDP lets startups focus on the data that matters most. This way, they can make smart decisions without wasting time and money. With a good BDP, startups can gather key data that supports their main goals. This helps them use AI tools to get useful insights, making it easier to adjust to what the market and customers need. It also helps everyone in the team understand data better without needing a lot of training or fancy systems. Another great thing about a BDP is that it encourages testing and learning. Startups can keep improving how they collect and analyze data. This trial-and-error approach supports new ideas and creativity, which is crucial for getting started in business. Plus, by using cloud services and free tools, startups can save money while still using powerful AI tools.
References
Kauffman, R. J., & Walden, E. A. (2021). “The Role of Data in Startup Growth: A Framework for Leveraging Data Analytics.” IEEE Transactions on Engineering Management.
Choudhury, P., & Kauffman, R. J. (2020). “Digital Transformation in the Era of Big Data and AI: Strategies for Startups.” Journal of Business Research.
Marr, B. (2016). Big Data in Practice: How 45 Successful Companies Used Big Data Analytics to Deliver Extraordinary Results. Wiley.
Ries, E. (2011). The Lean Startup: How Today’s Entrepreneurs Use Continuous Innovation to Create Radically Successful Businesses. Crown Business.
Osterwalder, A., & Pigneur, Y. (2010). Business Model Generation: A Handbook for Visionaries, Game Changers, and Challengers. Wiley.